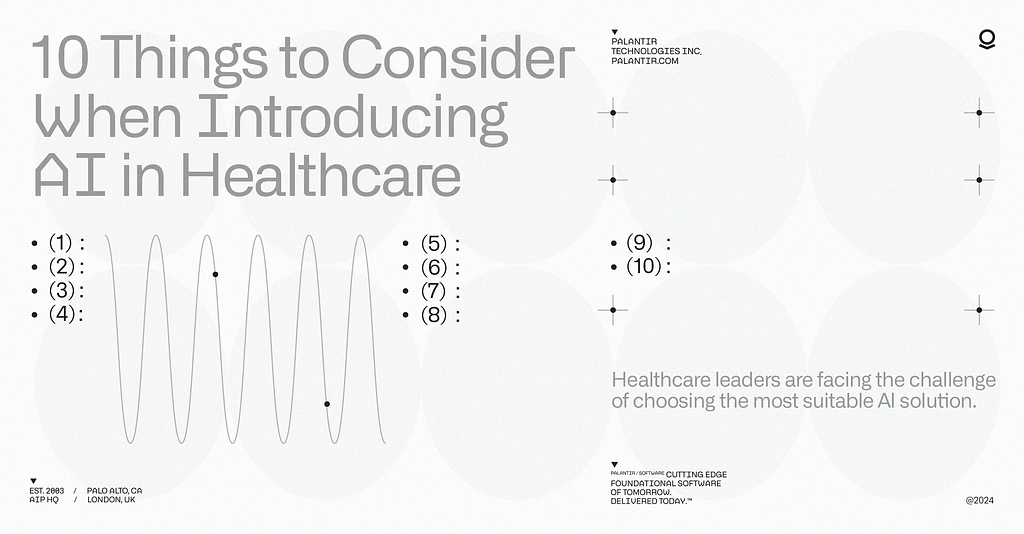
In the age of AI, healthcare leaders face the challenge of choosing the most suitable AI solution from a vast array of vendor options. In this blog post — drawing on insights from Palantir’s Artificial Intelligence Platform (AIP) for enhancing healthcare processes — we present 10 essential questions for healthcare leaders to consider when evaluating software that integrates AI into critical-path workflows, so that leaders can identify the solution most likely to bring about successful transformation.
1. What do you hope to gain by introducing AI?
AI has many applications. For an individual or organization, it might be workload efficiency gains, quality assessments, augment knowledge, compliance adherence, or complete automation. Determining key goals can help identify the appropriate subject matter experts (SMEs) and frameworks that should be used to guide and assess the AI system’s performance.
2. What level of accuracy does the AI have to achieve to be useful?
AI augmentation doesn’t always need to produce the entirety of the answer, as long as it’s integrated effectively with human intervention. Introducing AI can transform workflows even without addressing every possible scenario. This can help to identify decision points where incorporating a human-in-the-loop can still enhance a person’s ability to perform tasks on a larger scale. Determining this threshold is crucial for establishing a productive workflow, as the best improvements can often happen after AI is employed in the field.
3. How and when does the AI-derived output need to be presented to humans?
When using AI to extract information or generate predictions, recommendations, or decisions, consider how that information is presented to humans. The human decision-makers need to know that the data originated from an AI, which could affect their assessment of the information. High-confidence AI workflows typically “show their work,” providing a clear basis for their recommendations, including direct quotes from source texts and indicators that flag AI-derived fields. It is also important to create a long-term workforce training plan on how to effectively use these tools. As initial experts transition to other roles, the tools must remain instructive and informative for healthcare professionals of tomorrow.
4. Does the AI solution classify as an FDA-regulated Software as a Medical Device (SaMD)?
If the solution is involved in Clinical Decision Support or patient care and does not rely solely on human decision-making, it could be classified as a medical device. This classification has implications regarding the application’s compliance and safety, as well as the speed at which it can be improved. To determine if the software is classified as a medical device, consult the FDA’s four criteria outlined in this document: Your Clinical Decision Support Software_ Is it a Device_.pdf
5. Are the AI tools leveraged by the software compliant with applicable data protection frameworks?
AI tools, such as OpenAI’s GPT models and Google’s Gemini, are commonly utilized (under the hood) by software that can be leveraged in a manner that is generally compliant with legal frameworks such as HIPAA and GDPR. However, these tools are often not inherently compliant with your particular requirements, so it’s important to check that any third-party software adheres to the relevant security controls and practices that satisfy these frameworks. For instance, in HIPAA-regulated environments, the software provider should (among other things) be covered under a Business Associate Agreement (BAA). Appropriate provisions should also be included in any relevant data processing agreements with the software vendor. In cases where further restrictions are necessary, such as geo-restriction, these factors should be assessed as well and flowed down through the software supply chain.
6. Is it safe to leverage AI with Protected Health Information (PHI)?
In addition to regulatory compliance baselines, such as encryption in transit and at rest and basic security controls, it is important to understand if the data is being used for model training, whether the inputs could be stored or returned outside the context of the current prompt, or if the trained models could be applied in any context beyond their intended use. The ideal usage scenario involves zero retention outside of your control plane of prompts and responses, or the employment of a targeted model specifically designed for the intended use case(s). With these protections, it can be safe to leverage AI with these kinds of sensitive datasets.
7. Will there be an initial period of evaluation and iterative improvement?
After the AI is introduced, determine whether there will be a designated assessment period to evaluate its accuracy and tailor it to the unique nuances of the environment. What will the expected format be and how will SMEs be able to support it? A top-notch AI tool ought to be iterative — adapting to fit the environment — and may require changes to inputs and outputs for peak performance. These iterative enhancements should be tightly integrated with the production solution.
8. How will you validate and measure success?
Generative AI and predictive models often necessitate different testing methods compared to structured logic that anticipates precise outcomes. With generative AI, it is common for the same inputs to produce similar but varied outputs between runs. Establishing a robust validation plan to test AI accuracy is important. Testing strategies should include confidence thresholds (compelling the AI to offer contextual proof or refrain from providing an answer), incorporating feedback from humans-in-the-loop, employing traditional models for cross-verification (e.g., NLP examining contextual proof against source material), and analyzing a confusion matrix to assess the frequency of correct answers versus attempts. These strategies can enhance model accuracy and usefulness.
9. How will the AI address changes in the environment and model drift?
As AI models encounter varying inputs and shifting environments (e.g., seasonality, pandemics, holidays), their efficacy may be affected. To evaluate the usefulness of an AI model, it is important to understand how it is designed to adapt and prevent these common failure modes. Its capacity to adjust to fluctuations and maintain performance under varying conditions is an essential aspect of the AI’s long-term effectiveness.
10. How do you ensure you’re using the right AI model for the job?
The landscape of AI models is continuously evolving, with leading models changing rapidly. In early 2024, GPT models are at the forefront, with GPT-3.5 having been swiftly succeeded by GPT-4 and GPT-4o. Meanwhile, specialized models like Google’s MedLM were designed with an aim to perform more accurately in certain medical domains. Often, the best approach is to utilize a combination of models, as specific models excel at particular problem sets, while others may offer superior performance, lower costs, or reduced latency in different scenarios. The choice between fine-tuning or pre-training models can also influence the appropriateness of a model for a given task. Maintaining an open architecture that supports multi-modal problem-solving approaches and flexibility within the evaluation framework is essential for incorporating new AI models as they emerge.
Get Started Today
At Palantir, we address these questions on Day One. Whether you’re an established AI organization with comprehensive frameworks for each of these considerations or just beginning to explore AI-powered workflows, Palantir’s adaptable Artificial Intelligence Platform (AIP) enables you to leverage AI in healthcare today. Our platform tackles the most critical healthcare challenges across operations, finance, and research. Some of these use cases include dynamic nurse staffing and scheduling, patient placement, revenue cycle management (RCM), cohorting, real-world evidence (RWE), population health, and can expand to cover any solution that involves large volumes of unstructured text or manual intervention.
Discover how you can get started today with Palantir for Hospitals, Palantir for Life Sciences, or Palantir for Federal Health.
10 Things to Consider When Introducing AI in Healthcare was originally published in Palantir Blog on Medium, where people are continuing the conversation by highlighting and responding to this story.