Editors Note: The following is a collaboration between authors from Palantir’s Product Development and Privacy & Civil Liberties (PCL) teams. It outlines how our latest model management capabilities incorporate the principles of responsible artificial intelligence so that Palantir Foundry users can effectively solve their most challenging problems.
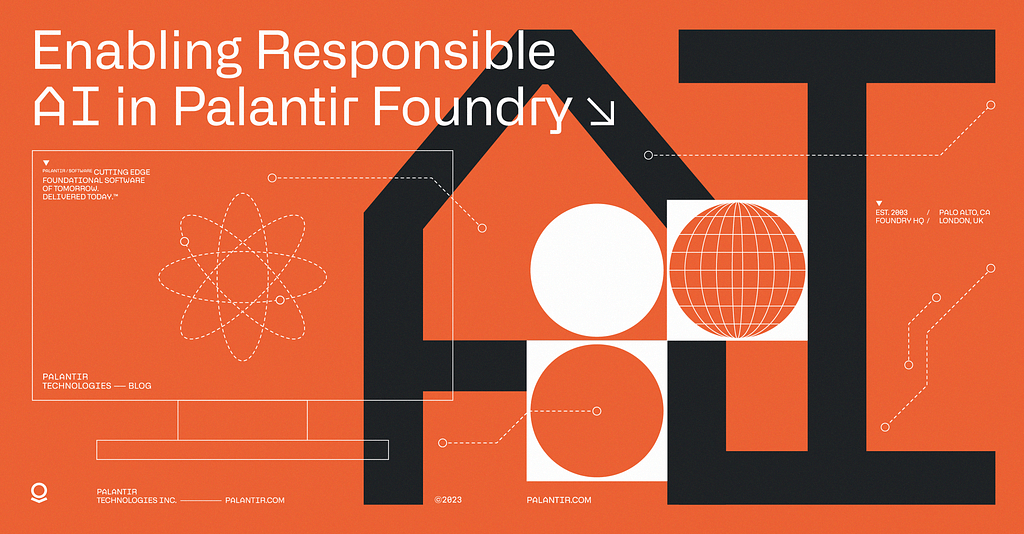
At Palantir, we’re proud to build mission-critical software for Artificial Intelligence (AI) and Machine Learning (ML). Foundry — our operating system for the modern organization — provides the infrastructure for users to develop, evaluate, deploy, and maintain AI/ML models to achieve their desired organizational outcomes.
From stabilizing consumer goods supply chains, to optimizing airplane manufacturing processes, and monitoring public health outbreaks across the globe, Foundry’s interoperable and extensible architecture has enabled data science teams worldwide to readily collaborate with their business and operational teams, enabling all stakeholders to create data-driven impact.
As we discussed in a previous data science blog post, using AI/ML for these important use cases demands software that spans the entire model lifecycle. Foundry’s first-class security and data quality tools enable users to develop AI/ML models, and by establishing a trustworthy data foundation, our software offers the connectivity and dynamic feedback loops that these teams need in order to sustain the effective use of models in practice.
Further to this, developing capabilities that facilitate the responsible use of artificial intelligence is an indispensable part of building industry-leading AI/ML capabilities. Here, we’ll share more about what responsible AI means at Palantir, and how Foundry’s latest model management and ModelOps capabilities enable organizations to address their most challenging problems.
Responsible AI at Palantir
At its core, our AI/ML product strategy centers around developing software that enables responsible AI use in both collaborative and operational settings. We believe that the term has many dimensions and includes considerations around AI safety, reliability, explainability, and governance. We’ve publicly advocated for a focused, problem-driven approach as well as the importance of robust data governance to AI/ML in multiple forums.
We believe that the tenets of responsible AI are not just limited to model development and use but have considerations throughout the entire model lifecycle. For example, developing reliable AI/ML solutions requires tools for the management and curation of high-quality data. These considerations extend beyond model deployment alone and include how end-users interact with their AI outputs and how they can use feedback loops for iteration, monitoring, and long-term maintenance.
Incorporating responsible AI principles in our software is also a core part of our commitment to privacy and civil liberties. Building this kind of software means recognizing that AI is not the solution to every problem and that a model for one problem will not always be a solution to others. A model’s intended use should be clearly and transparently scoped to specific business or operational problems.
Moreover, the challenges of using AI for mission-critical problems span a variety of domains and require expertise from a diverse breadth of disciplines. Building AI solutions should therefore be an interdisciplinary process where engineers, domain-experts, data scientists, compliance teams, and other relevant stakeholders work together to ensure the solution represents the specialized demands and requirements of the intended field of application. The values of responsible AI shape how we build our software, and in turn, they enable our customers to use AI/ML solutions in Foundry for their most critical problems.
Model Management in Foundry
Building on the platform’s robust security and data governance tools, Foundry’s model management capabilities are designed to encourage users to incorporate responsible AI principles throughout a model’s lifecycle. We have recently released product capabilities that improve the testing and evaluation ecosystem through no-code and low-code interfaces. We encourage you to read more about these here.
Problem-first modeling
In Foundry, orienting around the “operational problem” that models are trying to solve is at the heart of this new model management infrastructure. Foundry offers many tools for a data-first and exploratory approach to model experimentation, but for mission-critical use-cases, AI/ML applications need to be scoped to a specific problem. We have deliberately built modeling objectives to focus model development, evaluation, and deployment around well-defined problems.
The Modeling Objectives application enables users to define a problem, develop candidate models as solutions to these challenges, perform large-scale testing and evaluation, deploy models in many modalities to both staging and production applications, and then monitor them to enable faster iteration.
Specifying the modeling problem from the outset enables collaborators to better understand — and test for — the application and context for which the models are intended. This also provides greater insight into inadvertent reuse or repurposing of models. Modeling objectives provide a flexible yet structured framework that presents an opportunity to streamline model development and deployment by collecting key datasets, identifying stakeholders, and creating a testing and evaluation plan before their development begins.
These objectives also transparently communicate state about a particular AI/ML solution — from model development to testing, to deployment and further post-deployment actions like monitoring and upgrades. This enables users to be more intentional, responsible, and effective in how they use AI to address their organization’s operational challenges.
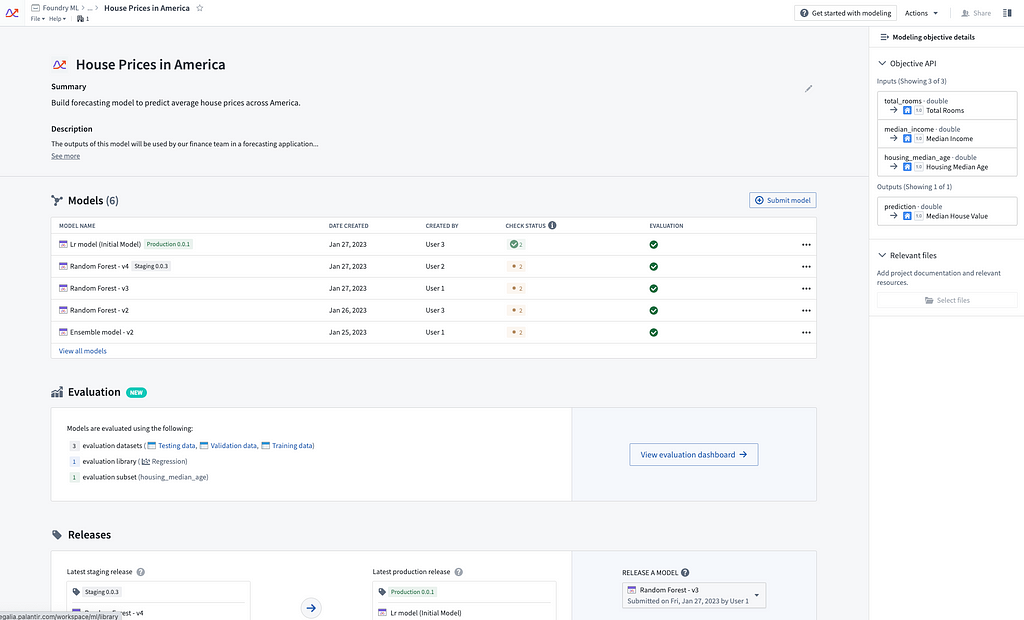
Deep integrations for security and governance
Data protection, governance, and security are core components of Palantir Foundry and are especially important for AI/ML. AI solutions must be traceable, auditable, and governable in order to be used effectively and responsibly. To facilitate this, Foundry’s model management infrastructure integrates deeply with the platform’s robust capabilities for versioning, branching, lineage, and access control.
Users can submit a model version to an objective and propose that model as a candidate solution for the problem defined in that objective. When submitting a model, users are encouraged to fill out metadata about the submission which becomes part of its permanent record. Project stakeholders and collaborators can use this to better understand the details of each submission and create a system of record that catalogs all future models for a particular modeling problem. With Data Lineage, they can also quickly see the provenance of every model that is submitted to an objective, revealing not only the models themselves, but also their training and testing data and what sources those datasets originally came from.
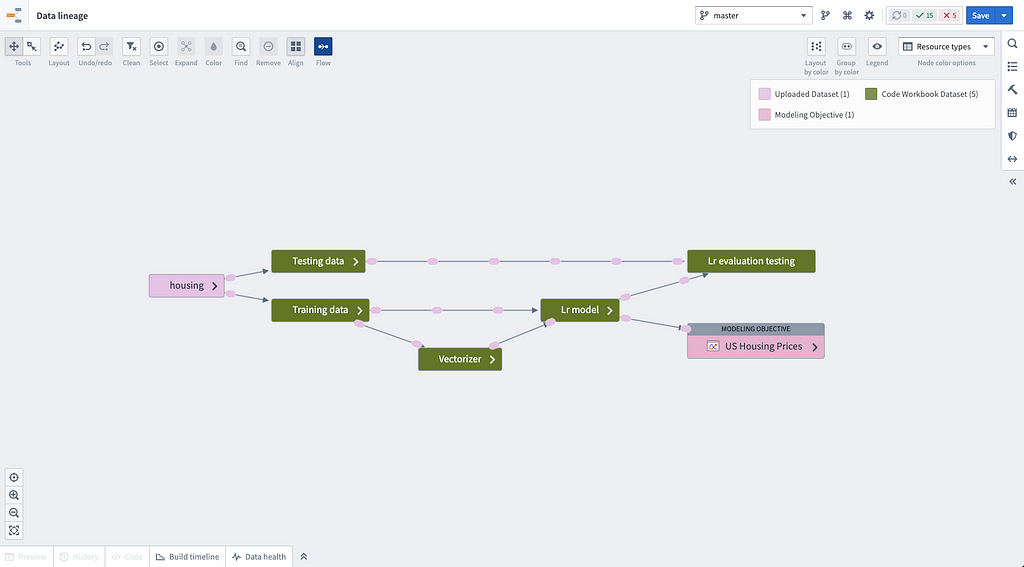
Foundry’s model management infrastructure natively integrates with the platform’s security primitives for access controls. This enables multiple model developers, evaluators, and other stakeholders to work together on the same modeling problem, while maintaining strict security and governance controls.
Robust testing and evaluation capabilities
Testing and evaluation (T&E) is one of the most critical steps in any model’s lifecycle. During T&E, subject matter experts, data scientists, and other business stakeholders determine whether a model is both effective and efficient for any given modeling problem. For example, models may need to be evaluated quantitatively and qualitatively, assessed for bias and fairness concerns, and checked against organizational requirements before they can be deployed to applications in production environments. That’s why we have released a new suite of capabilities to facilitate more effective and thorough T&E in Foundry.
Foundry now offers evaluation libraries for common AI/ML problems as a part of the Modeling Objectives application. The availability and native integration of these libraries within Foundry’s model management infrastructure enable users to quickly produce well-known, quantitative metrics in a point-and-click fashion for common modeling problems, all without having to dive into any technical implementation.
We’ve also included a framework for users to write their own custom evaluation libraries. Libraries authored in this framework benefit from the same UI-driven workflow and integration with modeling objectives. This extends the power of the integrated evaluation framework to more advanced modeling problems or context-specific use cases.
Building on the evaluation library integrations, we’ve also added the ability to easily evaluate models across subsets of data. This lets users quickly and exhaustively compute metrics to identify areas of model weakness that might otherwise go undetected if only computing aggregate metrics. Evaluating models on subsets can more easily surface bias or fairness concerns that affect only a portion of the model’s expected data distribution. Users can also configure their T&E workflows to run automatically on all candidate models proposed for a problem in order to build a T&E procedure that is both systematic and consistent.
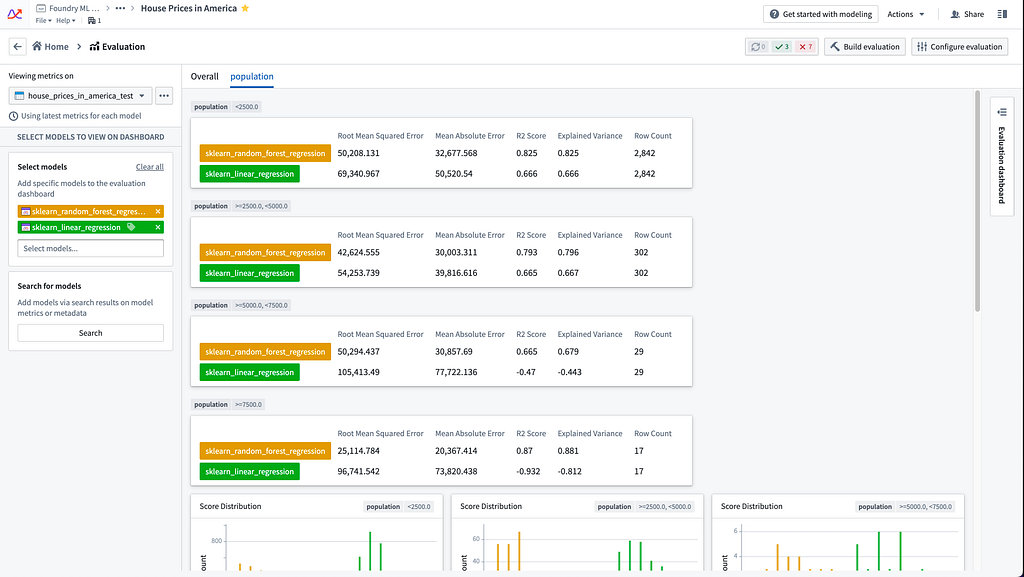
We also recognize that not all T&E procedures are quantitative. Therefore, checks in modeling objectives help keep track of certain pre-release tasks that might need to get done as part of the T&E process before a model can be released.
Looking ahead
Modeling objectives and the T&E suite are just some of the latest capabilities to encourage responsible AI in Foundry, and we continue to invest in new capabilities for effective model management. From the tools that facilitate robust model evaluation across domains, to mechanisms for seamless model release and rollback in production settings, our model management offering will always focus on empowering our customers to use their AI/ML solutions effectively, easily, and responsibly for their organization’s most challenging problems.
Visit our Artificial Intelligence and Machine Learning webpage to learn about AI/ML in Foundry and new improvements to our model management offering.
Authors
Arnav Jagasia, Privacy and Civil Liberties Engineering Lead
Angela McNeal, Head of AI/ML Product, Foundry
Courtney Bowman, Global Director of Privacy and Civil Liberties Engineering
Nick Weatherburn, Product Manager, AI/ML
Enabling Responsible AI in Palantir Foundry was originally published in Palantir Blog on Medium, where people are continuing the conversation by highlighting and responding to this story.